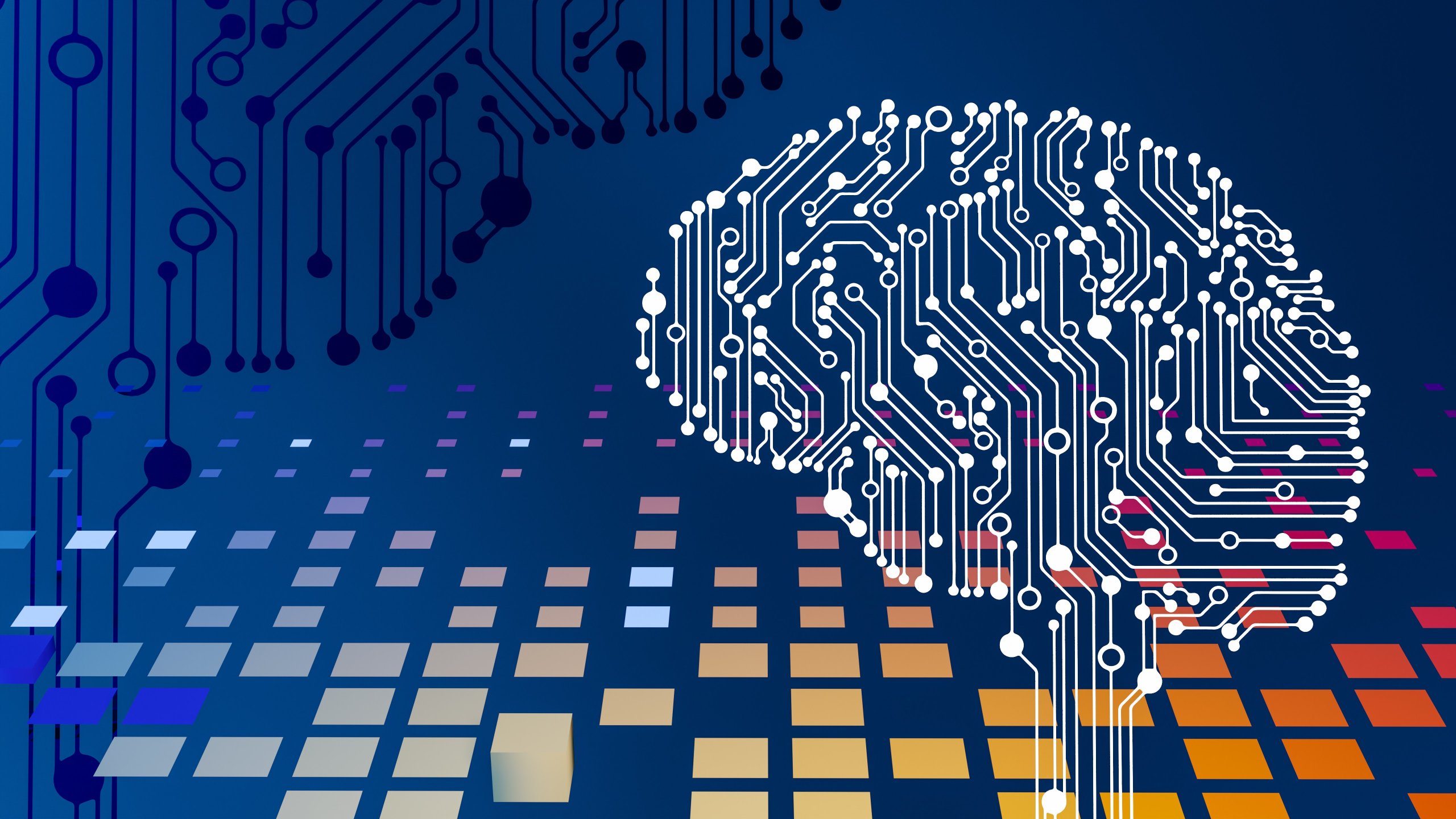
The integration of artificial neural networks and their variations is marking a new era in materials science research, especially in multiscale modelling and simulation
Machine learning and deep learning techniques are revolutionizing problem-solving in various fields, including materials science. Artificial neural networks (ANNs), including their variations as convolutional neural networks (CNNs) and recurrent neural networks (RNNs), have become one of the most effective machine learning approaches for applications in different computational materials science research topics, says Dr. Tae-Yeon Kim, Associate Professor of Civil Infrastructure and Environmental Engineering.
Dr. Kim collaborated with researchers from University of Iowa, Northwestern University, and the University of Luxembourg, to share insights to these machine learning techniques in the materials science community and promote their applications in research. Their results were published in Advances in Applied Mechanics, a top 1% journal for computational mechanics.
Advanced materials, which combine key properties, encompass multifunctional composites with enhanced capabilities. However, understanding these complex materials requires accurate estimates of physical properties — now possible through numerical modelling and simulations. Traditional computational methods have been used to study physical phenomena at various scales but the rising use of machine learning in materials science has helped to accelerate the discovery of new materials and improve material designs.
ANNs are computing algorithms inspired by biological neural networks. They are not programmed with rules but rather trained by considering examples related to various tasks. They have a higher capacity for progressive learning than other traditional machine learning models, mainly due to the potentially large number of parameters which can be tuned to progressively build more accurate models.
The research team’s paper offers a comprehensive overview of how these neural networks are being applied to some of the most advanced topics in material science, such as multiscale modelling, solving inverse problems, material image processing, and history-dependent constitutive modelling.
A key highlight of this research is a case study focused on metal-ceramic composite materials. In this study, the team meticulously modelled and simulated these materials at the microscale to create a rich dataset. This dataset then became the foundation for developing and training neural networks, which were tasked with predicting material failures at the macroscale. This approach not only demonstrates the practical application of ANNs in materials science but also underscores the potential of neural networks in bridging the gap between microscale observations and macroscale behaviors.
As computer technology continues to evolve, machine learning algorithms are expected to significantly enhance scientific understanding across various disciplines. This is particularly true for materials discovery and design, where the potential for innovation is vast.
Jade Sterling
Science Writer
17 January 2024